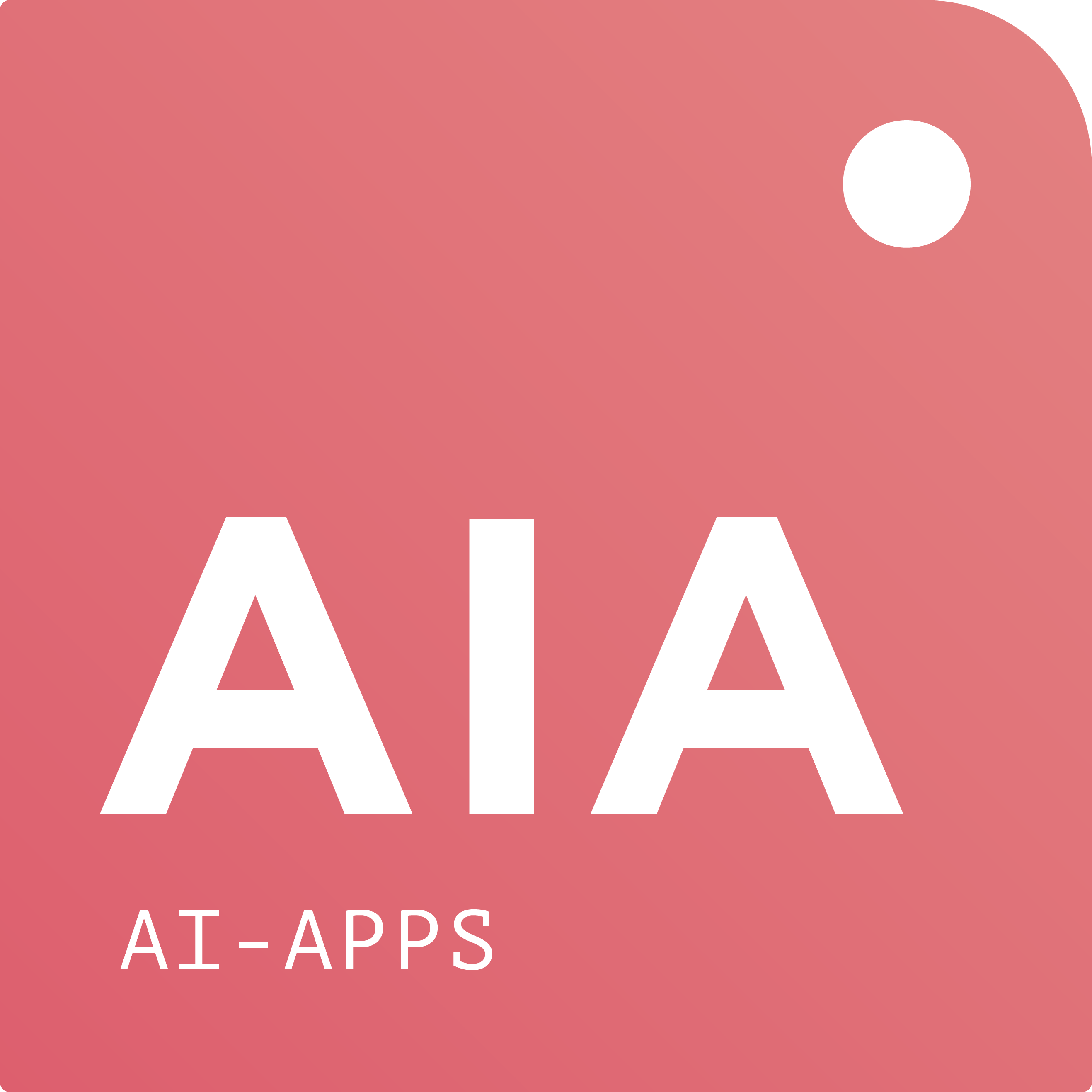
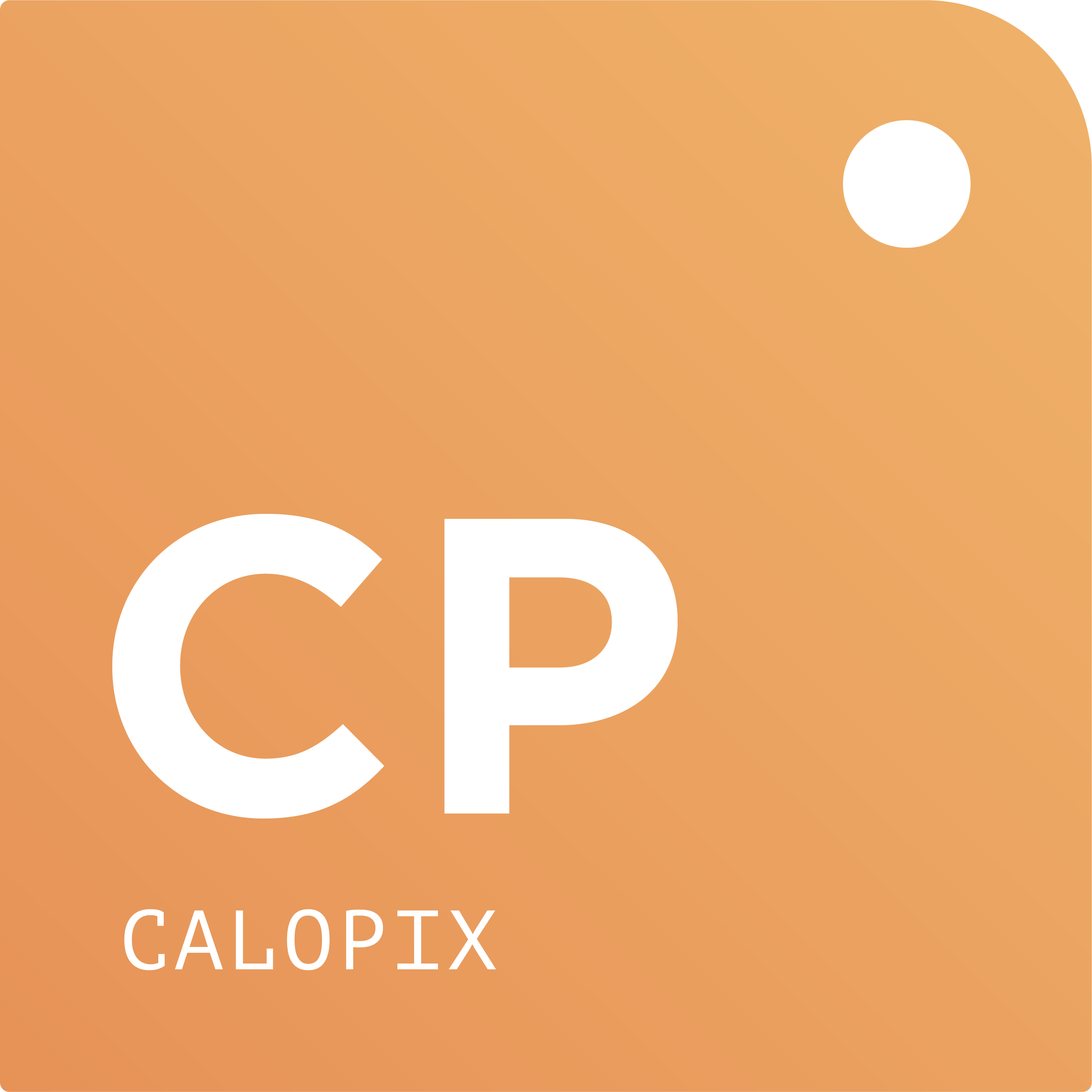
Nos solutions IA
Chez Tribun Health, notre engagement est de vous offrir le catalogue le plus complet de solutions avancées d'intelligence artificielle pour faciliter l'optimisation du flux de travail, ainsi que le diagnostic et le pronostic des pathologies.
Nous élargissons continuellement notre offre d'IA par le développement d'algorithmes internes, l'intégration fluide d'algorithmes de partenaires, la mise à disposition d'une API ouverte et la personnalisation d'algorithmes adaptés à vos besoins spécifiques.
Nous nous efforçons de proposer des options flexibles et d'offrir aux pathologistes des outils innovants qui améliorent la précision, l'efficacité et les résultats pour les patients.